Personalized social shopping represents a transformative shift in the e-commerce landscape. It leverages the power of social media and data analysis to create uniquely tailored shopping experiences for each individual user. Unlike traditional e-commerce, which often presents a generic catalog, personalized social shopping anticipates needs and preferences, offering relevant product recommendations and fostering a sense of community among like-minded shoppers.
This approach utilizes sophisticated algorithms that analyze vast amounts of data, including purchase history, social media interactions, and browsing behavior, to curate a highly personalized shopping journey. This results in increased customer engagement, higher conversion rates, and a more satisfying overall shopping experience. The integration of social influence further amplifies this effect, leveraging the power of recommendations and reviews from trusted peers and influencers.
Defining Personalized Social Shopping
Personalized social shopping represents a significant evolution from traditional e-commerce, moving beyond simple product listings and transactional interactions to create a deeply engaging and customized shopping experience integrated within the social fabric of online platforms. It leverages social interactions, user data, and advanced technologies to curate relevant product recommendations, foster community engagement, and ultimately drive sales. The key differentiator lies in its focus on individual preferences, social connections, and a more immersive shopping journey.Personalized social shopping differs from traditional e-commerce primarily in its emphasis on social influence and individual customization.
Traditional e-commerce focuses on product display and transactional efficiency, while personalized social shopping leverages social media interactions, user data analysis, and AI to tailor the shopping experience to each individual. This leads to increased engagement, higher conversion rates, and stronger brand loyalty.
Key Features of Personalized Social Shopping Experiences
Several key features distinguish personalized social shopping experiences. These include highly targeted product recommendations based on individual browsing history, purchase behavior, social interactions, and expressed preferences. Real-time personalized product feeds, dynamic pricing influenced by social demand and user behavior, and interactive social features like live shopping events and influencer collaborations all contribute to a more engaging and effective shopping experience.
The integration of social media platforms into the shopping journey itself, enabling seamless sharing and discovery, is another critical aspect. Finally, personalized social shopping frequently employs advanced analytics to track user behavior and optimize the overall experience.
Examples of Successful Personalized Social Shopping Platforms and Their Strategies
Several platforms exemplify the successful implementation of personalized social shopping strategies. For instance, Instagram Shopping allows influencers to tag products directly within their posts, creating a seamless shopping experience within the social media platform. This strategy leverages the power of influencer marketing and user trust to drive sales. Similarly, Pinterest’s visual discovery engine utilizes AI to curate personalized product recommendations based on users’ saved pins and interests, creating a highly targeted and engaging shopping journey.
These platforms successfully integrate shopping seamlessly into the social media experience, fostering organic engagement and conversion. TikTok Shop, with its short-form video format and integrated e-commerce features, allows for a highly dynamic and interactive shopping experience, often featuring live sales events and influencer collaborations. These platforms showcase the effectiveness of integrating social interaction with the shopping process.
Comparison of Personalized Social Shopping Models
The following table compares three different models of personalized social shopping: influencer-driven, community-based, and AI-powered.
Feature | Influencer-Driven | Community-Based | AI-Powered |
---|---|---|---|
Primary Driver | Influencer recommendations and endorsements | User-generated content and community feedback | Algorithmic personalization and predictive analytics |
Personalization Method | Targeted advertising and sponsored posts by relevant influencers | Product recommendations based on community preferences and reviews | Personalized product feeds, dynamic pricing, and targeted recommendations based on user data |
Engagement Strategy | Leveraging influencer’s audience and trust | Building a strong community around shared interests and product reviews | Providing a highly customized and efficient shopping experience |
Example Platforms | Instagram Shopping, TikTok Shop | Reddit communities focused on specific products, niche forums | Amazon’s personalized recommendations, Stitch Fix’s styling service |
The Role of Data and Algorithms
Personalized social shopping relies heavily on the sophisticated interplay of data collection and algorithmic processing to deliver tailored experiences. Understanding this relationship is crucial to appreciating both the potential and the limitations of this rapidly evolving retail model. The more data a platform gathers and the more refined its algorithms, the more accurately it can predict and cater to individual user preferences.
However, this power comes with ethical responsibilities that must be carefully considered.Data forms the bedrock of personalized social shopping. A wealth of information is collected to create detailed user profiles, enabling highly targeted recommendations. This data encompasses various aspects of user behavior and preferences.
Types of Data Used in Personalization
The effectiveness of personalized social shopping hinges on the comprehensive collection and analysis of diverse data points. This includes explicit data, such as information willingly provided by users during registration or profile creation (e.g., age, location, gender, interests), and implicit data, gleaned from user interactions with the platform. Examples of implicit data include purchase history (item types, brands, price points, purchase frequency), browsing behavior (products viewed, time spent on pages, abandoned carts), social media activity (likes, shares, comments on relevant posts and brands), and interactions with other users (e.g., following other users with similar tastes).
Combining these data sources provides a holistic view of the user, allowing for more accurate and relevant recommendations.
Algorithms for Product and Content Recommendation
Sophisticated algorithms are the engines that drive personalized recommendations. These algorithms analyze the vast datasets collected, identifying patterns and relationships to predict what products or content a user might be interested in. Common approaches include collaborative filtering (recommending items based on the preferences of similar users), content-based filtering (recommending items similar to those the user has previously interacted with), and hybrid approaches that combine both methods.
More advanced techniques incorporate machine learning models, such as deep learning neural networks, to capture complex relationships and improve prediction accuracy over time. For example, a deep learning model might analyze images of products alongside user reviews and purchase data to understand visual preferences and associate them with specific user demographics and purchasing behaviors.
Ethical Concerns Related to Data Collection and Usage
The power of personalized social shopping comes with significant ethical considerations. The extensive data collection raises concerns about user privacy and data security. Concerns include the potential for data breaches, unauthorized access to personal information, and the misuse of data for discriminatory purposes. Transparency in data collection practices and user consent are paramount. Furthermore, the potential for algorithmic bias needs careful attention.
Algorithms trained on biased data can perpetuate and amplify existing societal inequalities, leading to unfair or discriminatory outcomes. For instance, if a recommendation algorithm is trained primarily on data from a specific demographic, it may disproportionately recommend products to that group, neglecting the needs and preferences of other users. Robust mechanisms for detecting and mitigating bias are essential to ensure fairness and equity.
Hypothetical Product Recommendation Algorithm
A hypothetical algorithm could function as follows: First, a user profile is created based on explicitly provided data (age, location, interests) and implicit data (purchase history, browsing behavior, social media activity). This profile is represented as a vector of features, where each feature corresponds to a specific attribute (e.g., preference for a particular brand, price sensitivity, interest in sustainable products).
Next, product data is similarly represented as vectors of features. The algorithm calculates the similarity between the user profile vector and each product vector using a cosine similarity metric, for example. Products with the highest similarity scores are recommended to the user. Finally, social interactions are incorporated. If a user’s friends or followed users have purchased or interacted positively with a product, the algorithm boosts the product’s recommendation score, reflecting the influence of social networks on purchasing decisions.
This hybrid approach combines collaborative filtering with content-based filtering and incorporates social influence to provide a comprehensive and personalized recommendation system.
Social Influence and Community Building
Personalized social shopping thrives on the power of social influence and the creation of vibrant online communities. The recommendations, reviews, and shared experiences of others significantly impact individual purchasing decisions, making community engagement a crucial element for success in this model. Understanding how social influence operates and fostering strong communities are key to maximizing sales and building brand loyalty.
Social influence within personalized social shopping manifests in several ways. For example, seeing a friend purchase a particular item and subsequently posting a positive review on their social media feed can directly influence others in their network to make the same purchase. Similarly, influencer marketing, where brands collaborate with key opinion leaders on the platform, can drive significant sales through targeted recommendations and endorsements.
The visibility of products through user-generated content (UGC), such as photos and videos showcasing purchases, also acts as a powerful form of social proof, increasing the perceived value and desirability of those products.
Social Media Platform Effectiveness
The effectiveness of different social media platforms in driving personalized social shopping engagement varies significantly depending on the target audience and the brand’s strategy. Platforms like Instagram, with its strong visual focus and emphasis on lifestyle imagery, are particularly effective for showcasing products and building aspirational communities. Platforms like TikTok, known for its short-form video content and viral trends, offer opportunities for creative marketing campaigns and rapid product discovery.
Facebook, with its vast user base and robust targeting options, allows for highly personalized advertising and community building through groups. Ultimately, a multi-platform approach, tailored to the specific strengths of each platform and the preferences of the target demographic, often yields the best results.
Community Building Strategies
Building a strong sense of community around a personalized social shopping platform requires a multifaceted approach. Creating interactive features, such as live shopping events, Q&A sessions with brand representatives, and user-generated content contests, fosters engagement and encourages participation. Personalized recommendations and curated content based on individual user preferences create a more relevant and engaging experience. Building loyalty programs and offering exclusive benefits to active community members strengthens the sense of belonging and incentivizes continued engagement.
Responding promptly and authentically to user comments and feedback is crucial for building trust and fostering a positive community atmosphere.
Case Study: Sephora’s Social Commerce Success
Sephora successfully leverages social influence to boost sales through its personalized shopping experience. By integrating user reviews, influencer collaborations, and interactive features across its website and app, Sephora creates a dynamic and engaging shopping environment. Their “Sephora Squad” influencer program features diverse creators who authentically showcase products and interact with their followers, driving considerable traffic and sales. Sephora also actively utilizes user-generated content, featuring customer photos and videos on its platform, creating social proof and encouraging further engagement.
This multi-pronged approach, combining personalized recommendations with strong community building strategies and influencer marketing, has significantly contributed to Sephora’s success in the competitive beauty retail market. The integration of live shopping events further enhances this, allowing for real-time interaction and product demonstrations.
User Experience and Interface Design: Personalized Social Shopping
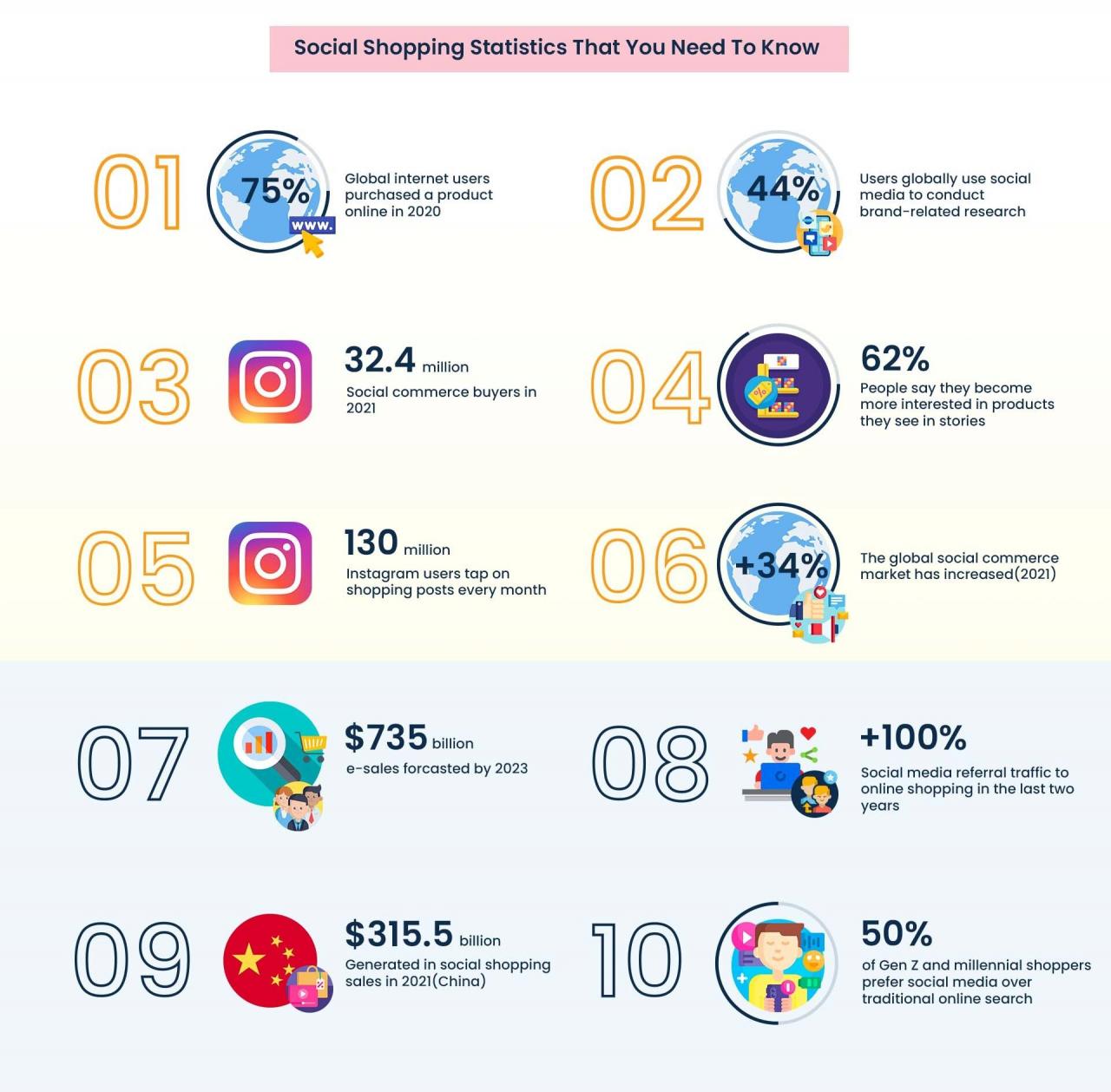
Source: vizury.com
A seamless and intuitive user experience is paramount for the success of any personalized social shopping platform. The ideal interface should effortlessly guide users through the process of discovering, sharing, and purchasing products within their personalized social network. This requires a careful consideration of visual design, navigation, and the integration of key features that enhance the overall shopping journey.The design should prioritize simplicity and clarity, avoiding overwhelming users with excessive information or complex interactions.
A clean, uncluttered layout, combined with intuitive navigation, ensures a smooth and enjoyable shopping experience. This is crucial for driving engagement and encouraging repeat visits.
Personalized Recommendations and Product Discovery
Personalized recommendations are the cornerstone of a successful personalized social shopping experience. The platform should leverage user data, purchase history, browsing behavior, and social interactions to provide highly relevant product suggestions. These recommendations should be presented in a visually appealing and easily digestible format, perhaps through visually rich carousels or curated collections tailored to individual preferences. For example, a user interested in sustainable fashion might see a curated collection of eco-friendly clothing items from brands they already follow or brands their friends have purchased from.
This approach leverages both algorithmic recommendations and social influence to maximize relevance and engagement.
Social Sharing and Community Building Features
The social aspect of the platform is crucial. Users should be able to easily share products they like with their friends and followers, fostering a sense of community and encouraging social discovery. This could include integrated social media sharing buttons, the ability to create wish lists and share them publicly, and the option to tag friends in product recommendations or reviews.
The design should seamlessly integrate these social features into the shopping experience, making them easily accessible and encouraging active participation. A prominent “Share” button on each product page, alongside a visually appealing feed displaying what friends have recently shared, would be ideal.
Visual Design Elements
Effective visual design plays a critical role in shaping the user experience. High-quality product photography, visually appealing layouts, and a consistent brand aesthetic are essential. The use of whitespace to avoid a cluttered feel, a clear and consistent color palette, and easy-to-read typography all contribute to a positive and engaging shopping environment. Furthermore, incorporating interactive elements, such as zoom functions for product images, 360° views, and video demonstrations, enhances the overall experience and allows users to engage more deeply with products before making a purchase.
Example: Personalized Social Shopping Product Page Mock-up
Imagine a product page for a stylish handbag. At the top, a large, high-resolution image of the handbag is displayed, with a zoom function allowing for detailed examination. Below the image, a concise and compelling product description highlights key features and benefits. Next, a section showcasing similar products recommended based on the user’s past purchases and social interactions is presented.
Below this, user reviews and ratings are displayed, encouraging social proof and building trust. Finally, a prominent “Add to Cart” button and integrated social sharing buttons (Facebook, Instagram, etc.) are located near the bottom, making the purchase process seamless and facilitating social sharing. The entire page maintains a clean and uncluttered layout, using a consistent color palette and high-quality imagery to create a visually appealing and user-friendly experience.
The page incorporates a section where users can see what their friends have purchased, promoting social influence and community engagement.
Marketing and Monetization Strategies
Successfully launching and sustaining a personalized social shopping platform requires a robust marketing strategy coupled with a well-defined monetization model. The right approach will attract users, build a loyal community, and generate revenue, ensuring the platform’s long-term viability. This section explores various marketing and monetization options, along with a sample marketing plan.
Marketing Strategies for Personalized Social Shopping Platforms
Effective marketing for a personalized social shopping platform hinges on highlighting its unique value proposition: a curated, personalized shopping experience tailored to individual preferences and social connections. Strategies should emphasize the convenience, discovery, and community aspects.
- Influencer Marketing: Partnering with relevant social media influencers to promote the platform and its features to their engaged audiences can significantly boost brand awareness and drive user acquisition. For example, collaborations with fashion or beauty influencers could introduce the platform to a highly targeted demographic.
- Content Marketing: Creating high-quality, engaging content (blog posts, articles, videos) around topics relevant to the platform’s niche (e.g., style guides, product reviews, shopping tips) can attract organic traffic and establish the platform as a trusted resource.
- Social Media Marketing: Utilizing various social media platforms (Instagram, TikTok, Pinterest, Facebook) to run targeted advertising campaigns and engage with potential users is crucial. This includes creating visually appealing content showcasing the platform’s personalized features and community aspect.
- Search Engine Optimization (): Optimizing the platform’s website and content for relevant s will improve its visibility in search engine results, attracting organic traffic from users actively searching for personalized shopping experiences.
- Email Marketing: Building an email list and sending targeted email campaigns to promote new features, exclusive deals, and personalized product recommendations can nurture user engagement and drive sales.
Monetization Models for Personalized Social Shopping Platforms
Several monetization models can be employed, either individually or in combination, to generate revenue from a personalized social shopping platform. The optimal approach depends on the platform’s target audience, features, and overall business strategy.
- Commission-Based Model: Earning a commission on each sale made through the platform is a common and effective monetization strategy. This involves partnering with retailers and earning a percentage of each transaction facilitated by the platform.
- Subscription-Based Model: Offering premium features or exclusive access to certain content or benefits through a subscription model can generate recurring revenue. This could include early access to sales, personalized styling advice, or exclusive discounts.
- Advertising Model: Displaying targeted advertisements to users based on their preferences and browsing history can generate revenue. This approach requires careful consideration to avoid overwhelming users with excessive ads and maintain a positive user experience.
- Affiliate Marketing: Partnering with other businesses or brands to promote their products or services in exchange for a commission on sales generated through referral links can provide additional revenue streams.
Comparison of Monetization Approaches, Personalized social shopping
Monetization Model | Advantages | Disadvantages |
---|---|---|
Commission-Based | Directly tied to sales, scalable, relatively simple to implement | Dependent on sales volume, can be challenging to negotiate favorable commission rates with retailers |
Subscription-Based | Predictable recurring revenue, fosters user loyalty, allows for premium features | Requires users to pay upfront, can limit accessibility, requires strong value proposition |
Advertising-Based | Potential for high revenue, relatively low effort to implement | Can be intrusive to users, requires careful management to avoid negatively impacting user experience, revenue can be unpredictable |
Marketing Plan for a New Personalized Social Shopping Platform
This plan Artikels a phased approach to launching a new personalized social shopping platform focusing on sustainable growth.
Target Audiences:
- Fashion-conscious millennials and Gen Z: Highly active on social media, interested in personalized experiences and unique style.
- Busy professionals seeking convenience: Value time-saving solutions and personalized recommendations for efficient shopping.
Marketing Channels:
- Phase 1 (Pre-launch): Influencer marketing campaigns on Instagram and TikTok, building anticipation through social media contests and giveaways.
- Phase 2 (Launch): Targeted advertising campaigns on social media, optimization for relevant s, email marketing to pre-registered users.
- Phase 3 (Post-launch): Content marketing (blog posts, style guides), community building initiatives, affiliate marketing partnerships.
Future Trends and Challenges
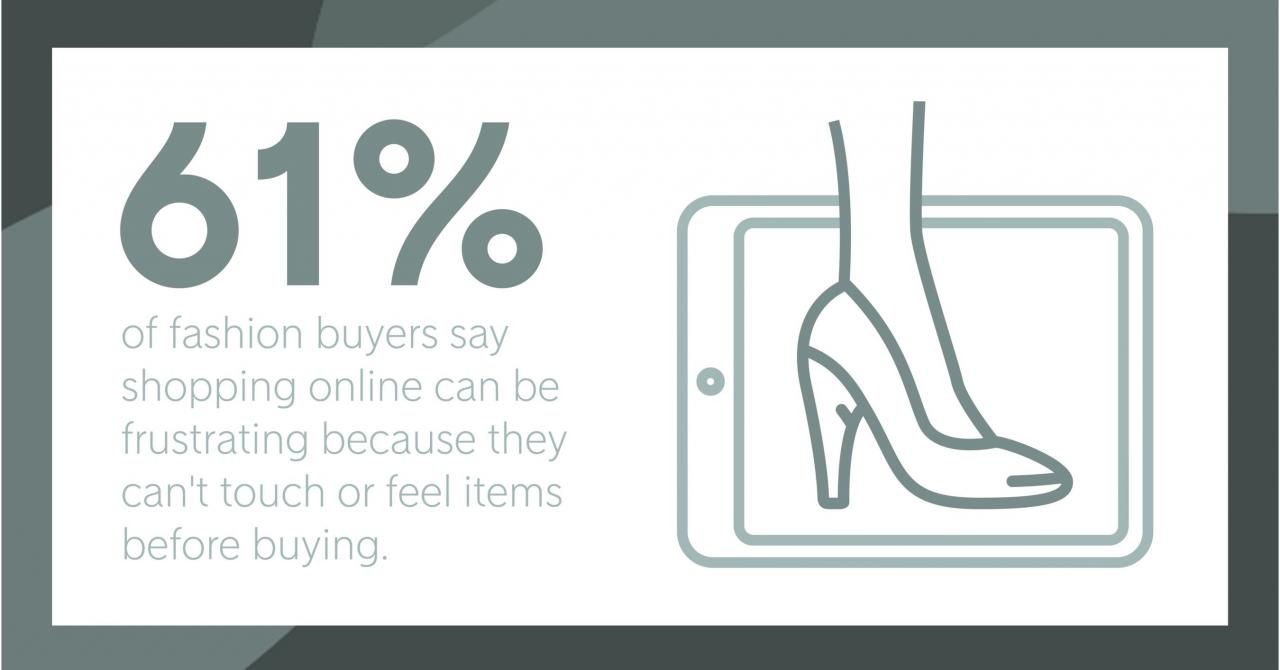
Source: prnewswire.com
Personalized social shopping, while offering exciting opportunities, faces a complex landscape of emerging trends and significant challenges. The rapid evolution of technology and shifting consumer expectations necessitate a proactive approach to navigate this dynamic environment and capitalize on future growth. Success will depend on adapting to these trends and effectively addressing the inherent challenges.
The future of personalized social shopping hinges on the successful integration of innovative technologies and the proactive management of potential risks. Failure to adapt to evolving consumer preferences and address concerns regarding data privacy could severely limit the growth and long-term viability of these platforms.
Emerging Technologies and Their Integration
The integration of augmented reality (AR) and virtual reality (VR) technologies presents significant opportunities for enhancing the personalized social shopping experience. AR can allow users to virtually “try on” clothes or visualize furniture in their homes before purchasing, significantly reducing purchase uncertainty. VR, on the other hand, could create immersive shopping experiences, allowing users to explore virtual stores and interact with products in a more engaging way.
For example, a cosmetics company could use AR to allow users to virtually try on different shades of lipstick, while a furniture retailer might leverage VR to let users explore a virtual showroom and design their ideal living space. This level of engagement can significantly increase conversion rates and customer satisfaction.
Data Privacy Concerns and Mitigation Strategies
Personalized social shopping relies heavily on data collection and analysis to understand consumer preferences and tailor recommendations. However, this raises significant concerns about data privacy and security. Users are increasingly wary of sharing their personal information, and breaches of data privacy can have severe reputational and legal consequences. Robust data encryption, transparent data handling policies, and user control over data usage are crucial to building trust and maintaining compliance with regulations like GDPR and CCPA.
Implementing strong data anonymization techniques and utilizing federated learning models, which train algorithms on decentralized data without directly accessing sensitive information, are also vital solutions.
Competition from Established E-commerce Giants
Personalized social shopping platforms face stiff competition from established e-commerce giants like Amazon and Alibaba, which possess vast resources, established customer bases, and sophisticated logistical networks. To compete effectively, smaller platforms need to differentiate themselves through unique value propositions, such as highly curated product selections, strong community building, and exceptional customer service. Focusing on niche markets or specific demographics can also help to carve out a competitive advantage.
For example, a platform specializing in sustainable fashion or ethically sourced products could attract a loyal customer base that is less susceptible to the allure of larger, more generalist platforms.
A Vision for the Future of Personalized Social Shopping
The future of personalized social shopping involves a seamless blend of technology, community, and ethical practices. We can envision platforms that leverage AI-powered recommendation systems to anticipate consumer needs, providing highly personalized shopping experiences that are both convenient and enjoyable. The integration of blockchain technology could enhance transparency and trust by providing secure and verifiable product provenance. Furthermore, a stronger emphasis on sustainable practices and ethical sourcing will become increasingly important, appealing to the growing number of environmentally and socially conscious consumers.
Ultimately, the most successful platforms will be those that prioritize user privacy, foster strong community engagement, and offer unique and valuable shopping experiences that resonate with their target audiences.
Epilogue
In conclusion, personalized social shopping is poised to redefine the future of e-commerce. By seamlessly blending social interaction with tailored product recommendations, it offers a more engaging and effective shopping experience than traditional models. While challenges remain, particularly regarding data privacy and ethical considerations, the potential benefits—increased customer loyalty, higher sales conversion, and the fostering of vibrant online communities—make it a compelling and rapidly evolving area of commerce.
Question & Answer Hub
What are the potential downsides of personalized social shopping?
Potential downsides include concerns about data privacy, the potential for filter bubbles and algorithmic bias, and the risk of creating overly targeted advertising that can feel intrusive to consumers.
How can businesses ensure ethical data usage in personalized social shopping?
Businesses should prioritize transparency, obtain explicit user consent, implement robust data security measures, and provide users with control over their data and privacy settings. Adherence to relevant data protection regulations is crucial.
What role do influencers play in personalized social shopping?
Influencers serve as trusted sources of product recommendations and can significantly impact purchasing decisions. Their authentic engagement and connection with their followers make them highly effective advocates for brands and products within this model.
How does personalized social shopping differ from targeted advertising?
While both utilize data to tailor their approach, personalized social shopping goes beyond simple ad targeting. It integrates recommendations seamlessly into the shopping experience, leveraging social interaction and community aspects to enhance engagement and build loyalty.