AI-powered social commerce is revolutionizing the way businesses interact with consumers. It leverages artificial intelligence to personalize the shopping experience, enhance customer service, and optimize marketing efforts, ultimately driving increased engagement and sales. This transformative approach blends the social interaction of platforms like Facebook and Instagram with the power of AI-driven insights to create a more efficient and customer-centric commerce ecosystem.
The integration of AI algorithms allows for highly targeted recommendations, automated customer support, and sophisticated fraud detection, setting a new standard for online retail.
This exploration delves into the core components of AI-powered social commerce, examining its key differences from traditional methods and showcasing successful implementations. We will analyze AI’s role in personalization, customer service, marketing, and fraud detection, while also forecasting future trends and addressing potential ethical considerations. The goal is to provide a comprehensive understanding of this rapidly evolving field and its impact on the future of retail.
Defining AI-Powered Social Commerce
AI-powered social commerce represents a significant evolution in the way businesses interact with and sell to consumers online. It leverages artificial intelligence to enhance various aspects of the social commerce experience, ultimately driving greater efficiency, personalization, and sales conversion. This approach goes beyond simply using social media platforms for selling; it integrates AI capabilities directly into the commerce process.AI-powered social commerce platforms incorporate several key components working in synergy.
These components are crucial for delivering a seamless and intelligent shopping experience.
AI-powered social commerce is rapidly transforming how businesses interact with customers, offering personalized experiences and streamlined purchasing. A prime example of this synergy is seen in the evolution of visual platforms like Pinterest, which are increasingly integrated with e-commerce functionalities. For a deeper dive into this integration, check out this insightful article on Pinterest for e-commerce , which highlights how these platforms are leveraging AI to enhance the shopping journey.
Ultimately, this convergence promises a more intuitive and efficient future for AI-powered social commerce.
Core Components of AI-Powered Social Commerce Platforms
The core components typically include sophisticated recommendation engines, personalized marketing tools, advanced analytics dashboards, chatbot interfaces for customer service, and automated order fulfillment systems. These components are interwoven to create a dynamic and responsive ecosystem. Recommendation engines, for example, use machine learning algorithms to analyze user data and suggest relevant products, while personalized marketing tools tailor advertisements and promotions to individual customer preferences.
The analytics dashboards provide real-time insights into customer behavior and campaign performance, enabling data-driven decision-making. Chatbots offer instant customer support, addressing queries and resolving issues promptly. Finally, automated order fulfillment systems streamline the entire process from order placement to delivery.
Key Differences Between Traditional and AI-Driven Social Commerce
Traditional social commerce largely relies on manual processes and generic marketing strategies. While it utilizes social media platforms for sales, the level of personalization and automation is significantly limited. In contrast, AI-driven social commerce leverages machine learning and deep learning algorithms to automate tasks, personalize the customer journey, and optimize sales funnels. This leads to improved customer experience, increased conversion rates, and more efficient resource allocation.
The key difference lies in the level of intelligence and automation incorporated into the platform. Traditional approaches are largely reactive, while AI-driven approaches are proactive and predictive.
Examples of Successful AI-Powered Social Commerce Implementations
Several companies have successfully integrated AI into their social commerce strategies, achieving impressive results. The following table showcases some notable examples.
AI-powered social commerce is rapidly transforming how we shop, leveraging data to personalize recommendations and streamline the buying process. A prime example of this integration is seen in the innovative features offered by TikTok, as detailed in this insightful article on TikTok shopping features. Ultimately, these advancements highlight the potential of AI to create a more engaging and efficient shopping experience within social media platforms.
Company | Platform | AI Application | Key Results |
---|---|---|---|
Sephora | Instagram, Facebook | AI-powered product recommendations, personalized beauty consultations via chatbot | Increased engagement, higher conversion rates, improved customer satisfaction |
Nike | Various social media platforms | AI-driven targeted advertising, personalized shopping experiences based on user preferences and past purchases | Improved brand awareness, enhanced customer loyalty, increased sales |
Starbucks | Instagram, Facebook | AI-powered chatbot for ordering and customer support, personalized offers and promotions based on location and purchase history | Streamlined ordering process, improved customer service, increased sales |
Amazon | Various social media platforms, Amazon’s own platforms | AI-powered product recommendations, personalized marketing campaigns, fraud detection, inventory management | Increased sales, improved customer experience, reduced operational costs |
AI’s Role in Personalization and Recommendations: AI-powered Social Commerce
AI is revolutionizing social commerce by enabling highly personalized shopping experiences. This goes beyond simply suggesting similar products; it involves understanding individual customer preferences, behaviors, and needs to deliver tailored product recommendations and offers, ultimately increasing engagement and driving sales. This deep level of personalization is made possible through sophisticated AI algorithms that analyze vast amounts of data.AI algorithms personalize the shopping experience by analyzing various data points to create detailed user profiles.
This includes browsing history, purchase history, social media activity, demographics, and even real-time interactions within the social commerce platform. By identifying patterns and preferences, AI can predict what a user might be interested in purchasing next, proactively suggesting relevant products and offers. This targeted approach fosters a more engaging and satisfying shopping journey.
AI-Driven Recommendation Systems in Social Commerce
Several different AI-driven recommendation systems are employed in social commerce platforms. These systems leverage various techniques to provide relevant product suggestions. The choice of system often depends on the platform’s specific goals and the available data.
- Collaborative Filtering: This system analyzes the purchasing and browsing behavior of similar users to recommend products that those users have liked or purchased. For example, if User A frequently buys sustainable fashion and User B has similar buying patterns, the system will recommend similar sustainable fashion items to User B.
- Content-Based Filtering: This approach focuses on the characteristics of the products themselves. If a user purchases a specific type of running shoe, the system will recommend other running shoes with similar features or from the same brand. This is effective for users with well-defined preferences.
- Hybrid Recommendation Systems: Many platforms utilize a hybrid approach, combining collaborative and content-based filtering to leverage the strengths of both. This can provide a more comprehensive and accurate recommendation set.
- Knowledge-Based Systems: These systems use explicit user preferences and product attributes to create recommendations. A user might specify their preferred color, size, and brand, and the system will filter products accordingly. This is especially useful for users who know exactly what they want.
Impact of Personalized Recommendations on Customer Engagement and Conversion Rates
Personalized recommendations significantly influence customer engagement and conversion rates within social commerce. By presenting relevant products, these systems increase the likelihood of purchase.
- Increased Engagement: Personalized recommendations make the shopping experience more relevant and engaging. When users see products they are actually interested in, they spend more time browsing and interacting with the platform.
- Higher Conversion Rates: By presenting products users are likely to purchase, personalized recommendations directly increase conversion rates. This translates to higher sales and revenue for the business.
- Improved Customer Satisfaction: A more personalized experience leads to increased customer satisfaction. When users feel understood and valued, they are more likely to become loyal customers.
- Reduced Cart Abandonment: By suggesting complementary products or reminding users of items left in their cart, personalized recommendations can reduce cart abandonment rates.
AI-Driven Customer Service and Support
AI is rapidly transforming customer service within social commerce, offering businesses unprecedented opportunities to enhance customer experience and efficiency. The integration of artificial intelligence allows for faster response times, personalized interactions, and valuable data-driven insights into customer sentiment. This leads to improved customer satisfaction, increased sales, and a stronger brand reputation.AI chatbots and virtual assistants are at the forefront of this transformation.
They provide immediate support, answering frequently asked questions, resolving simple issues, and guiding customers through the purchasing process. This frees up human agents to focus on more complex problems, leading to greater overall efficiency and reduced customer wait times. The use of AI also extends to analyzing vast amounts of customer data to identify trends and patterns, allowing businesses to proactively address potential issues and improve their offerings.
AI-powered social commerce is revolutionizing the retail landscape, offering highly targeted advertising and improved customer experiences. A key element of this advancement is the rise of personalized recommendations, exemplified by the sophisticated strategies detailed in this insightful article on Personalized social shopping. Ultimately, this focus on individual preferences fuels the growth and efficiency of AI-driven social commerce platforms.
AI Chatbots and Virtual Assistants in Social Commerce Customer Service
AI-powered chatbots and virtual assistants significantly enhance customer service within social commerce platforms by providing readily available, 24/7 support. These tools can handle a wide range of tasks, from answering basic product inquiries and providing order tracking information to assisting with returns and exchanges. They are programmed to understand natural language, allowing for more natural and intuitive interactions with customers.
For example, a chatbot on a fashion retailer’s Instagram page could instantly answer questions about sizing, shipping costs, or available colors, significantly reducing response times and improving customer satisfaction. Advanced chatbots can even learn from past interactions to continuously improve their accuracy and efficiency. This personalized and immediate service fosters customer loyalty and improves the overall shopping experience.
AI-Driven Sentiment Analysis of Customer Feedback
Sentiment analysis, a powerful application of AI, enables social commerce platforms to understand the emotional tone and overall sentiment expressed in customer feedback across various social media channels. This involves using natural language processing (NLP) techniques to analyze text data, such as customer reviews, comments, and social media posts, to determine whether the sentiment is positive, negative, or neutral. For instance, a social listening tool might detect an increase in negative comments regarding a specific product’s quality.
This early warning system allows businesses to quickly address issues, prevent negative word-of-mouth, and protect their brand reputation. The insights gained from sentiment analysis are crucial for improving products, services, and overall customer experience. For example, identifying a recurring negative sentiment regarding slow shipping times can prompt the business to investigate its logistics processes and implement improvements.
Hypothetical AI-Powered Customer Service System for Social Commerce
A comprehensive AI-powered customer service system for a social commerce platform would integrate various AI technologies to provide a seamless and efficient customer support experience.
- 24/7 Multi-Channel Support: The system would offer instant support across multiple channels, including social media platforms (Facebook, Instagram, Twitter), in-app chat, and email.
- Intelligent Chatbots: Advanced chatbots capable of understanding complex queries, providing personalized recommendations, and escalating complex issues to human agents.
- Proactive Customer Support: The system would proactively identify and address potential customer issues based on sentiment analysis of social media conversations and customer feedback.
- Automated Ticket Routing: AI would automatically route customer inquiries to the appropriate department or agent based on the nature of the problem.
- Sentiment Analysis Dashboard: A real-time dashboard visualizing customer sentiment across various channels, allowing businesses to quickly identify and respond to negative feedback.
- Personalized Recommendations: The system could integrate with the recommendation engine to provide tailored product suggestions based on customer interactions and past purchases.
- Knowledge Base Integration: The system would be integrated with a comprehensive knowledge base, allowing chatbots to access and provide accurate information quickly.
- Performance Monitoring and Analytics: Detailed analytics on customer service performance, including response times, resolution rates, and customer satisfaction scores.
Impact of AI on Social Commerce Marketing
Artificial intelligence is revolutionizing social commerce marketing, enabling businesses to reach their target audiences with unprecedented precision and efficiency. By leveraging AI’s analytical capabilities and predictive power, marketers can optimize campaigns, personalize experiences, and ultimately drive higher conversion rates. This section explores the significant impact of AI on various aspects of social commerce marketing.AI significantly enhances the effectiveness of targeted advertising and influencer marketing within the social commerce landscape.
It allows for a level of personalization previously unimaginable, moving beyond broad demographic targeting to micro-segmentation based on individual consumer behavior and preferences.
Targeted Advertising and Influencer Marketing with AI
AI-powered platforms analyze vast datasets of user information, including browsing history, purchase behavior, social media interactions, and demographics, to create highly targeted advertising campaigns. For example, an AI system might identify users who have shown interest in a particular product category on multiple platforms and then serve them personalized ads showcasing relevant items. This approach significantly improves ad relevance and click-through rates.
Similarly, AI assists in influencer marketing by identifying influencers whose audience aligns perfectly with a brand’s target demographic and whose engagement levels suggest a high likelihood of campaign success. AI algorithms can analyze influencer performance metrics, such as engagement rate, audience demographics, and past campaign results, to select the most effective partners for a given marketing campaign. This ensures that marketing budgets are allocated strategically to influencers with a proven track record of delivering results.
AI-powered social commerce is rapidly transforming how businesses engage with consumers, leveraging data to personalize experiences and optimize sales funnels. A key component of this evolution involves harnessing the power of video platforms, and a prime example is the burgeoning trend of YouTube social selling , which allows for direct interaction and immediate purchasing opportunities. Ultimately, integrating these video-driven strategies strengthens the overall efficacy of AI-powered social commerce initiatives.
AI-Driven Optimization of Social Media Campaigns
AI plays a crucial role in optimizing social media campaigns for better reach and engagement. Through real-time data analysis, AI algorithms can identify which content resonates most effectively with the target audience, at what times, and on which platforms. This enables marketers to dynamically adjust their campaigns, optimizing content, timing, and targeting parameters to maximize reach and engagement. For instance, an AI system might analyze the performance of different ad creatives and automatically allocate more budget to the highest-performing ads, while simultaneously reducing spending on underperforming ones.
Furthermore, AI can identify and predict trends, allowing marketers to adapt their strategies proactively to capitalize on emerging opportunities and avoid potential pitfalls. Predictive analytics can also help anticipate customer behavior, enabling preemptive actions to enhance engagement and customer satisfaction.
Comparison of AI-Driven and Traditional Social Media Marketing
The following table compares the effectiveness of AI-driven marketing strategies with traditional social media marketing methods across key metrics:
Metric | AI-Driven Marketing | Traditional Marketing |
---|---|---|
Cost | Potentially higher initial investment in AI tools, but potentially lower overall cost due to improved efficiency and targeting. | Generally lower initial investment, but potentially higher overall cost due to wasted ad spend and lower conversion rates. |
Reach | Wider and more targeted reach due to advanced segmentation and personalization capabilities. | Limited reach, often relying on broad demographic targeting with lower precision. |
Engagement | Higher engagement rates due to personalized content and timely delivery. | Lower engagement rates due to generic content and less precise targeting. |
Conversion Rates | Significantly higher conversion rates due to improved targeting and personalization. | Lower conversion rates due to less effective targeting and generic messaging. |
AI and Fraud Detection in Social Commerce
The rise of social commerce has unfortunately created fertile ground for various fraudulent activities. The ease of creating accounts, the reliance on user-generated content, and the often less stringent verification processes compared to traditional e-commerce platforms make social commerce particularly vulnerable. Effective fraud detection is therefore crucial for maintaining trust and ensuring the long-term viability of these platforms.
AI offers a powerful toolset to address this challenge.AI algorithms are proving invaluable in identifying and preventing fraudulent activities within social commerce. The sheer volume of data generated on these platforms – user interactions, transactions, reviews, and content – makes manual fraud detection impractical. AI’s ability to process this data at scale and identify subtle patterns indicative of fraud provides a significant advantage.
Specifically, machine learning models can be trained to recognize anomalies in user behavior, product listings, and review patterns, flagging suspicious activities for further investigation.
Common Types of Social Commerce Fraud
Social commerce platforms face a range of fraudulent activities. These include the creation of fake accounts used for spamming, manipulating reviews, or engaging in coordinated attacks to inflate product popularity. Another significant issue is fake product reviews, either positive or negative, designed to manipulate consumer perception and influence purchasing decisions. Additionally, fraudulent transactions, including chargebacks and stolen credit card information, pose a serious threat.
Finally, the use of bots to automate interactions, such as liking posts or following accounts, is prevalent and can be used to artificially boost engagement metrics.
AI-Based Fraud Detection Mechanisms
AI algorithms leverage various techniques to detect and prevent fraudulent activities. Machine learning models, such as neural networks and random forests, are trained on vast datasets of historical transactions and user behavior. These models identify patterns and anomalies that are characteristic of fraudulent activities. For example, an algorithm might flag an account that suddenly generates an unusually high number of reviews for various products or one that exhibits erratic purchase patterns.
Natural Language Processing (NLP) techniques can analyze the text of reviews, identifying inconsistencies or patterns suggestive of fake reviews. Anomaly detection algorithms can pinpoint unusual spikes in activity, such as a sudden surge in follows or likes from a particular account, which could indicate bot activity.
Conceptual Model of an AI-Based Fraud Detection System
A robust AI-based fraud detection system requires a multi-faceted approach. The following Artikels a conceptual model for such a system:
- Data Ingestion: Collecting data from various sources, including user profiles, transactions, reviews, and social media interactions.
- Data Preprocessing: Cleaning and transforming the raw data to make it suitable for analysis. This includes handling missing values, removing duplicates, and converting data into a usable format.
- Feature Engineering: Creating relevant features from the preprocessed data that can be used by the machine learning models. Examples include the number of reviews per user, the sentiment of reviews, and the frequency of transactions.
- Model Training: Training machine learning models on a labeled dataset of fraudulent and legitimate activities. This allows the models to learn to distinguish between the two.
- Fraud Detection: Using the trained models to analyze real-time data and identify potentially fraudulent activities.
- Alerting and Investigation: Generating alerts for suspicious activities and providing tools for human investigators to review and verify the flagged instances.
- Model Monitoring and Retraining: Continuously monitoring the performance of the models and retraining them periodically to adapt to evolving fraud techniques.
Future Trends in AI-Powered Social Commerce
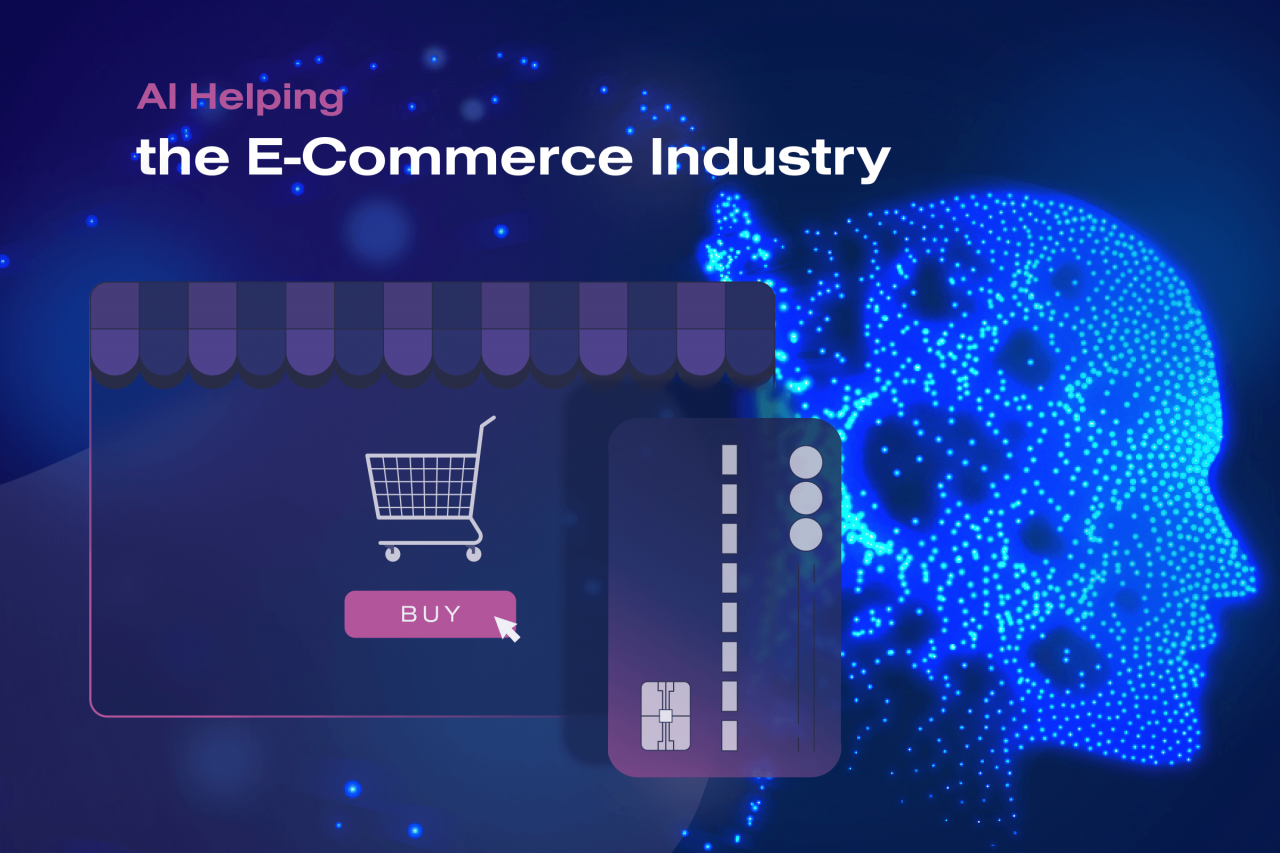
Source: soloway.tech
The next five years promise a dramatic reshaping of social commerce, driven by increasingly sophisticated AI applications. We can expect to see AI move beyond its current role of enhancing user experience to becoming a core engine driving business strategy and operational efficiency within social platforms. This evolution will bring both exciting opportunities and significant challenges.AI’s role in social commerce will evolve towards more proactive and predictive capabilities.
Instead of simply reacting to user behavior, AI will anticipate needs and preferences, offering personalized experiences that are both highly relevant and surprisingly intuitive. This will involve a deeper integration of AI across all aspects of the social commerce ecosystem, from product discovery and purchase to customer service and fraud prevention.
AI-Driven Hyper-Personalization and Predictive Analytics
The future of AI in social commerce will be defined by hyper-personalization. AI algorithms will go beyond basic recommendations, analyzing vast amounts of data – including purchase history, browsing behavior, social interactions, and even real-time contextual information – to create uniquely tailored shopping experiences for each individual. For example, an AI system might anticipate a user’s need for a new pair of running shoes based on their recent fitness tracker data and social media posts, proactively suggesting relevant products before the user even expresses a need.
This level of predictive analytics will also improve inventory management and supply chain optimization for businesses. Imagine a scenario where AI accurately predicts demand spikes based on upcoming events or social media trends, allowing businesses to avoid stockouts and optimize their logistics.
Enhanced Conversational Commerce and AI-Powered Customer Support
AI-powered chatbots and virtual assistants will become even more sophisticated, capable of handling increasingly complex customer queries and transactions. These systems will leverage natural language processing (NLP) and machine learning (ML) to understand nuances in customer communication, providing personalized support that feels human-like. Beyond basic order tracking and returns, these AI assistants could help customers style outfits based on their preferences, offer personalized styling advice, or even provide real-time assistance during the checkout process.
This evolution will lead to a significant improvement in customer satisfaction and loyalty. Consider a system that proactively addresses a potential shipping delay, offering alternative solutions before the customer even notices the issue.
AI-powered social commerce leverages data to personalize shopping experiences, but building trust remains crucial. A key element in this trust-building process is the effective use of social proof, as detailed in this insightful article on Social proof in e-commerce. Ultimately, AI can enhance the presentation and impact of social proof, further boosting sales conversions within the social commerce ecosystem.
The Rise of AI-Driven Influencer Marketing and Content Creation, AI-powered social commerce
AI will play a crucial role in optimizing influencer marketing campaigns. Algorithms will identify the most effective influencers for specific products and target audiences, predicting campaign performance and maximizing return on investment. Furthermore, AI-powered tools will assist in content creation, generating personalized product descriptions, ad copy, and even social media posts tailored to specific influencer styles and audience preferences.
This will streamline the influencer marketing process, making it more efficient and effective. For example, an AI system could analyze an influencer’s past performance, audience demographics, and content style to suggest optimal posting times and hashtags, significantly improving engagement rates.
Challenges and Ethical Considerations
The increasing reliance on AI in social commerce presents several challenges and ethical considerations. Data privacy is paramount; robust measures must be in place to protect user data and ensure compliance with relevant regulations. Algorithmic bias is another concern; AI systems trained on biased data can perpetuate and even amplify existing inequalities. Transparency is crucial; users should understand how AI is being used to personalize their shopping experience and have the ability to control their data.
Finally, the potential for AI-driven manipulation and misinformation requires careful consideration and proactive mitigation strategies.
Projected Growth of AI in Social Commerce
Imagine a graph depicting the adoption rate of AI-powered features in social commerce over the next five years. The x-axis represents time (in years), while the y-axis represents the percentage of social commerce platforms integrating AI features. The graph would show a steep upward curve, starting from a relatively low baseline in 2024 and rapidly accelerating in the following years.
The curve would likely plateau around 80-90% adoption by 2028, indicating near-universal integration of AI in social commerce. Different colored lines could represent specific AI features (e.g., personalized recommendations, AI-powered chatbots, fraud detection) to illustrate their individual adoption rates and growth trajectories. The graph would visually communicate the significant and rapid growth anticipated in the adoption and utilization of AI in social commerce.
Final Summary
In conclusion, AI-powered social commerce represents a significant leap forward in the retail landscape. By harnessing the power of artificial intelligence, businesses can create more personalized, efficient, and secure shopping experiences. While challenges and ethical considerations exist, the potential benefits—from increased customer engagement and conversion rates to enhanced fraud detection—are undeniable. The future of social commerce is inextricably linked to the continued development and responsible implementation of AI, promising a more streamlined and customer-centric future for online retail.
General Inquiries
What are the main challenges in implementing AI-powered social commerce?
Challenges include data privacy concerns, the need for high-quality data, the cost of implementing AI systems, and the potential for algorithmic bias.
How can businesses measure the ROI of AI investments in social commerce?
ROI can be measured by tracking key metrics such as conversion rates, customer lifetime value, customer acquisition cost, and overall sales growth. A/B testing comparing AI-driven campaigns to traditional methods is also crucial.
What are some ethical considerations related to AI in social commerce?
Ethical concerns include potential biases in algorithms leading to unfair treatment of certain customer groups, data privacy violations, and the lack of transparency in AI decision-making processes.
How can businesses ensure data privacy while using AI in social commerce?
Businesses should adhere to data privacy regulations (like GDPR and CCPA), obtain explicit user consent for data collection, implement robust data security measures, and be transparent about how user data is used.